AI & ML
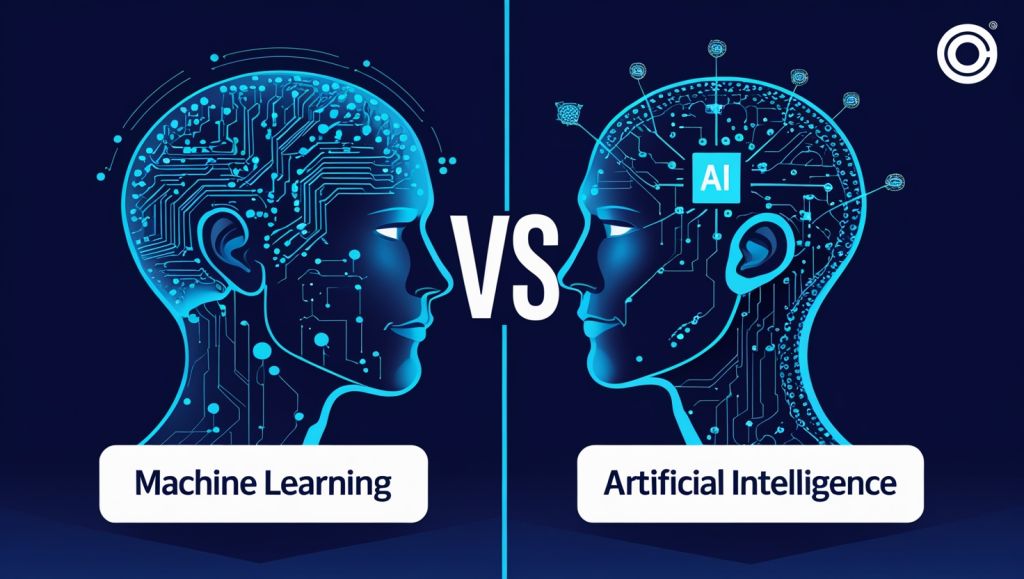
In a digital world, artificial intelligence and machine learning can serve as transformative technologies reshaping industries, economies, everyday life and AI can create systems capable of performing tasks that normally require human intelligence, such as reasoning, problem solving, cognition and language comprehension.Machine learning is an important subset of AI, which can empower systems to learn and improve from experience without explicit programming, and by analyzing a wide range of data sets, ML algorithms detect patterns, make predictions, and adapt over time. Three basic paradigms define ML:
Supervised learning, for example spam detection using labeled emails
Unsupervised learning, for example customer segmentation through clustering
Reinforcement learning, for example games that master AI through trial and reward
As AI and ML continue to change, we promise to our customers unprecedented efficiency and innovation, while also raising ethical questions about privacy, workforce impacts and These technologies advance healthcare diagnostic imaging, financial fraud detection, and personalized recommendations. Understanding these technologies is key to developing their capabilities and challenges in an increasingly intelligent world, and beginning during the Dartmouth conference, AI initially focused on symbolic methods and rule-based systems.
What is AI and ML?
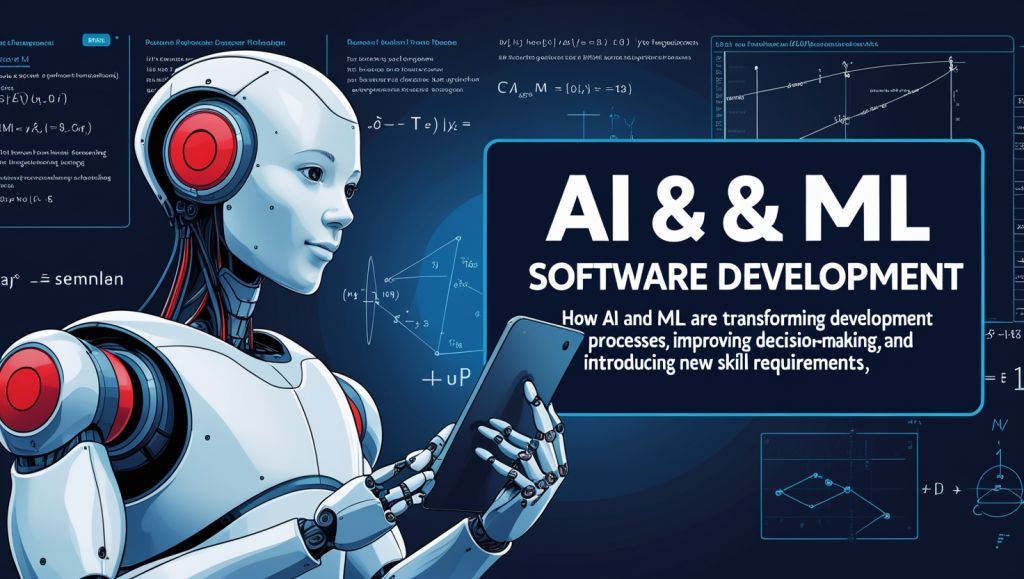
Artificial intelligence as the simulation of human intelligence in machines, enabling them to perform tasks such as decision making, problem solving, language processing, and cognition, on the other hand, machine learning is known as a fundamental subset of AI, focusing on developing algorithms that allow systems to learn from data, recognize patterns, and make decisions with human intervention.AI systems range from simple rule-based algorithms to sophisticated systems that mimic human perception, and instead of relying on clear programming, ML models improve their performance by exposure to new data.
AI and ML services we provide:
- Predictive analytics and decision making
- Natural Language Processing
- Computer Vision Solutions
- Custom AI/ML model development
- Process automation
- Deep Learning and Neural Networks
- AI/ML Consulting and Integration
Applications of AI and ML across industries
AI and ML are revolutionizing industries by automating, improving decision-making and opening up new opportunities and here are some applications in sectors:
Health care: AI analyzes medical images such as X-rays, MRIs to detect cancer, fractures or anomalies with human-level accuracy and ML models predict molecular interactions, speeding up drug development for example in the research of COVID-19 vaccines
Finance: ML identifies suspicious transactions by finding patterns in real-time data and AI models analyze market trends to execute high-frequency trading
Retail and e-commerce: ML personalized product recommendations for example Amazon, Netflix and AI forecasts demand to improve stock levels and reduce waste
Manufacturing: AI models streamline logistics, reduce costs or delays and Sensors + ML predict equipment failure, minimizing downtime
Agriculture: ML analyzes satellite images to identify threats early and AI predicts crop volume using weather and historical data
Transportation and Logistics: ML plans fuel-efficient routes for the fleet and AI systems reduce the crowd by analyzing real-time traffic data
Education: AI adapts coursework to students individual needs or pace and ML evaluates assignments and provides feedback
How AI and ML work
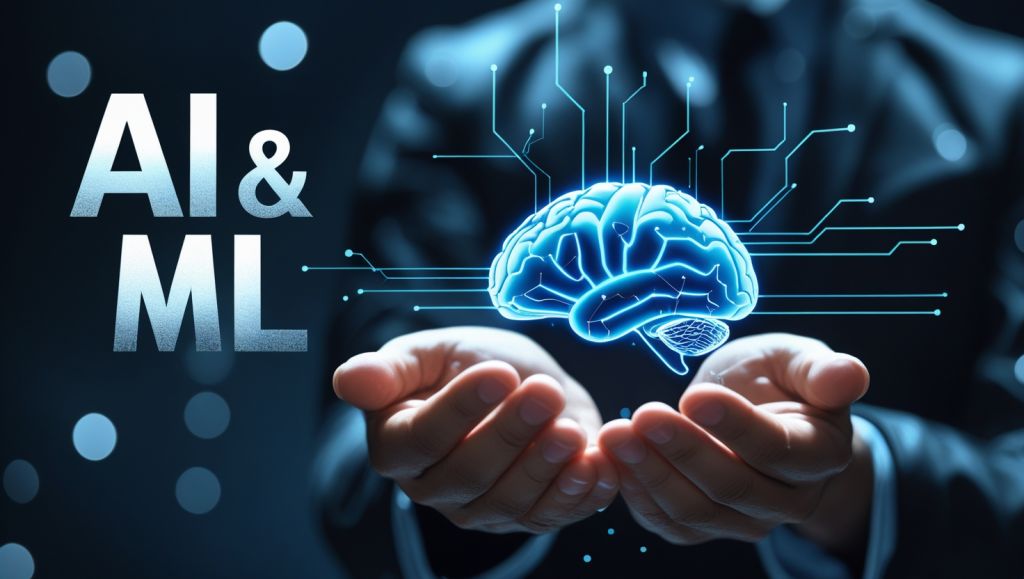
AI refers to creating systems capable of performing tasks that normally require human intelligence, such as decision-making, language understanding and visual recognition and AI can be principle-based, for example expert systems or adaptive, where machine learning comes into play and Machine learning as a subset of AI it enables systems to learn from data without explicit programming, so algorithms can be used to identify patterns, make decisions, improve over time, and ML is a key driver of modern AI innovations, there are following Process steps in ML:
- Data collection and preprocessing
- Model selection
- training
- Validation
- Testing and deployment.
Ethical Considerations in AI and ML
As AI and ML become more advanced, they raise many ethical concerns that need to be addressed to ensure responsible and fair use Many AI models, especially deep learning, act as “black boxes”, making it difficult to understand how they make decisions and the solution is to develop explainable AI methods to provide insight into decision making and here are some key ethical considerations:
- Transparency and clarity
- Privacy:
- Accountability and responsibility:
- Job displacement:
- Safety and security
Frequently Asked Questions
Q:1 What is the difference between Deep Learning vs ML?
Ans: Deep learning uses multilayered neural networks to analyze high-dimensional data such as images, speech and traditional ML, for example, often relies on simple models for decision trees for structured data.
Q:2 What are the real-world AI/ML applications?
Ans: Recommendation systems such as Netflix, Spotify, medical diagnostics such as x-rays and tumor detection in autonomous vehicles, fraud detection, and chatbots.
Q:3 How do ML models learn?
Ans: They analyze data to identify patterns using statistical methods, for example:
Supervised learning involves training a model on labeled data, for example detecting spam using spam or not spam emails and Unsupervised learning is a model that finds hidden patterns in unlabeled data for customer segmentation.
Q:4 What are the most common challenges in AI and ML?
Ans: Below are some of the challenges that users face and you have to keep in mind:
- Data quality and bias
- Interpretation of models
- Ethical concerns such as job displacement, fairness in decision making and
- High computational requirements
Conclusion
Artificial intelligence and machine learning are transformative technologies reshaping industries, economies or everyday life and While specialized AI tasks such as image recognition, predictive analytics and ML adaptive algorithms continue to evolve, enabling systems to learn and improve without human intervention. Looking ahead, advances in interpretable AI, quantum machine learning, and human-AI collaboration promise even greater possibilities and moreover By balancing innovation with ethical responsibility, society can leverage these tools to solve complex problems, foster creativity, and build a future where technology serves the collective advancement of humanity.